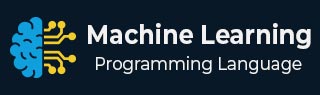
- Machine Learning Basics
- Machine Learning - Home
- Machine Learning - Getting Started
- Machine Learning - Basic Concepts
- Machine Learning - Python Libraries
- Machine Learning - Applications
- Machine Learning - Life Cycle
- Machine Learning - Required Skills
- Machine Learning - Implementation
- Machine Learning - Challenges & Common Issues
- Machine Learning - Limitations
- Machine Learning - Reallife Examples
- Machine Learning - Data Structure
- Machine Learning - Mathematics
- Machine Learning - Artificial Intelligence
- Machine Learning - Neural Networks
- Machine Learning - Deep Learning
- Machine Learning - Getting Datasets
- Machine Learning - Categorical Data
- Machine Learning - Data Loading
- Machine Learning - Data Understanding
- Machine Learning - Data Preparation
- Machine Learning - Models
- Machine Learning - Supervised
- Machine Learning - Unsupervised
- Machine Learning - Semi-supervised
- Machine Learning - Reinforcement
- Machine Learning - Supervised vs. Unsupervised
- Machine Learning Data Visualization
- Machine Learning - Data Visualization
- Machine Learning - Histograms
- Machine Learning - Density Plots
- Machine Learning - Box and Whisker Plots
- Machine Learning - Correlation Matrix Plots
- Machine Learning - Scatter Matrix Plots
- Statistics for Machine Learning
- Machine Learning - Statistics
- Machine Learning - Mean, Median, Mode
- Machine Learning - Standard Deviation
- Machine Learning - Percentiles
- Machine Learning - Data Distribution
- Machine Learning - Skewness and Kurtosis
- Machine Learning - Bias and Variance
- Machine Learning - Hypothesis
- Regression Analysis In ML
- Machine Learning - Regression Analysis
- Machine Learning - Linear Regression
- Machine Learning - Simple Linear Regression
- Machine Learning - Multiple Linear Regression
- Machine Learning - Polynomial Regression
- Classification Algorithms In ML
- Machine Learning - Classification Algorithms
- Machine Learning - Logistic Regression
- Machine Learning - K-Nearest Neighbors (KNN)
- Machine Learning - Naïve Bayes Algorithm
- Machine Learning - Decision Tree Algorithm
- Machine Learning - Support Vector Machine
- Machine Learning - Random Forest
- Machine Learning - Confusion Matrix
- Machine Learning - Stochastic Gradient Descent
- Clustering Algorithms In ML
- Machine Learning - Clustering Algorithms
- Machine Learning - Centroid-Based Clustering
- Machine Learning - K-Means Clustering
- Machine Learning - K-Medoids Clustering
- Machine Learning - Mean-Shift Clustering
- Machine Learning - Hierarchical Clustering
- Machine Learning - Density-Based Clustering
- Machine Learning - DBSCAN Clustering
- Machine Learning - OPTICS Clustering
- Machine Learning - HDBSCAN Clustering
- Machine Learning - BIRCH Clustering
- Machine Learning - Affinity Propagation
- Machine Learning - Distribution-Based Clustering
- Machine Learning - Agglomerative Clustering
- Dimensionality Reduction In ML
- Machine Learning - Dimensionality Reduction
- Machine Learning - Feature Selection
- Machine Learning - Feature Extraction
- Machine Learning - Backward Elimination
- Machine Learning - Forward Feature Construction
- Machine Learning - High Correlation Filter
- Machine Learning - Low Variance Filter
- Machine Learning - Missing Values Ratio
- Machine Learning - Principal Component Analysis
- Machine Learning Miscellaneous
- Machine Learning - Performance Metrics
- Machine Learning - Automatic Workflows
- Machine Learning - Boost Model Performance
- Machine Learning - Gradient Boosting
- Machine Learning - Bootstrap Aggregation (Bagging)
- Machine Learning - Cross Validation
- Machine Learning - AUC-ROC Curve
- Machine Learning - Grid Search
- Machine Learning - Data Scaling
- Machine Learning - Train and Test
- Machine Learning - Association Rules
- Machine Learning - Apriori Algorithm
- Machine Learning - Gaussian Discriminant Analysis
- Machine Learning - Cost Function
- Machine Learning - Bayes Theorem
- Machine Learning - Precision and Recall
- Machine Learning - Adversarial
- Machine Learning - Stacking
- Machine Learning - Epoch
- Machine Learning - Perceptron
- Machine Learning - Regularization
- Machine Learning - Overfitting
- Machine Learning - P-value
- Machine Learning - Entropy
- Machine Learning - MLOps
- Machine Learning - Data Leakage
- Machine Learning - Resources
- Machine Learning - Quick Guide
- Machine Learning - Useful Resources
- Machine Learning - Discussion
Machine Learning - Bias and Variance
Bias and variance are two important concepts in machine learning that describe the sources of error in a model's predictions. Bias refers to the error that results from oversimplifying the underlying relationship between the input features and the output variable, while variance refers to the error that results from being too sensitive to fluctuations in the training data.
In machine learning, we strive to minimize both bias and variance in order to build a model that can accurately predict on unseen data. A model with high bias may be too simplistic and underfit the training data, while a model with high variance may overfit the training data and fail to generalize to new data.
Example
Below is an implementation example in Python that illustrates how bias and variance can be analyzed using the Boston Housing dataset −
import numpy as np import pandas as pd from sklearn.datasets import load_boston boston = load_boston() X = boston.data y = boston.target from sklearn.model_selection import train_test_split X_train, X_test, y_train, y_test = train_test_split(X, y, test_size=0.2, random_state=42) from sklearn.linear_model import LinearRegression from sklearn.metrics import mean_squared_error lr = LinearRegression() lr.fit(X_train, y_train) train_preds = lr.predict(X_train) train_mse = mean_squared_error(y_train, train_preds) print("Training MSE:", train_mse) test_preds = lr.predict(X_test) test_mse = mean_squared_error(y_test, test_preds) print("Testing MSE:", test_mse)
Output
The output shows the training and testing mean squared errors (MSE) of the linear regression model. The training MSE is 21.64 and the testing MSE is 24.29, indicating that the model has a moderate level of bias and variance.
Training MSE: 21.641412753226312 Testing MSE: 24.291119474973456
Reducing Bias and Variance
To reduce bias, we can use more complex models that can capture non-linear relationships in the data.
Example
Let's try a polynomial regression model −
from sklearn.preprocessing import PolynomialFeatures poly = PolynomialFeatures(degree=2) X_train_poly = poly.fit_transform(X_train) X_test_poly = poly.transform(X_test) pr = LinearRegression() pr.fit(X_train_poly, y_train) train_preds = pr.predict(X_train_poly) train_mse = mean_squared_error(y_train, train_preds) print("Training MSE:", train_mse) test_preds = pr.predict(X_test_poly) test_mse = mean_squared_error(y_test, test_preds) print("Testing MSE:", test_mse)
Output
The output shows the training and testing MSE of the polynomial regression model with degree=2. The training MSE is 5.31 and the testing MSE is 14.18, indicating that the model has a lower bias but higher variance compared to the linear regression model.
Training MSE: 5.31446956670908 Testing MSE: 14.183558207567042
Example
To reduce variance, we can use regularization techniques such as ridge regression or lasso regression. In the following example, we will be using ridge regression −
from sklearn.linear_model import Ridge ridge = Ridge(alpha=1) ridge.fit(X_train_poly, y_train) train_preds = ridge.predict(X_train_poly) train_mse = mean_squared_error(y_train, train_preds) print("Training MSE:", train_mse) test_preds = ridge.predict(X_test_poly) test_mse = mean_squared_error(y_test, test_preds) print("Testing MSE:", test_mse)
Output
The output shows the training and testing MSE of the ridge regression model with alpha=1. The training MSE is 9.03 and the testing MSE is 13.88 compared to the polynomial regression model, indicating that the model has a lower variance but slightly higher bias.
Training MSE: 9.03220937860839 Testing MSE: 13.882093755326755
Example
We can further tune the hyperparameter alpha to find the optimal balance between bias and variance. Let's see an example −
from sklearn.model_selection import GridSearchCV param_grid = {'alpha': np.logspace(-3, 3, 7)} ridge_cv = GridSearchCV(Ridge(), param_grid, cv=5) ridge_cv.fit(X_train_poly, y_train) train_preds = ridge_cv.predict(X_train_poly) train_mse = mean_squared_error(y_train, train_preds) print("Training MSE:", train_mse) test_preds = ridge_cv.predict(X_test_poly) test_mse = mean_squared_error(y_test, test_preds) print("Testing MSE:", test_mse)
Output
The output shows the training and testing MSE of the ridge regression model with the optimal alpha value.
Training MSE: 8.326082686584716 Testing MSE: 12.873907256619141
The training MSE is 8.32 and the testing MSE is 12.87, indicating that the model has a good balance between bias and variance.
To Continue Learning Please Login