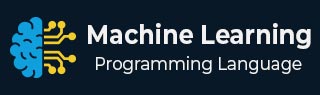
- Machine Learning Basics
- Machine Learning - Home
- Machine Learning - Getting Started
- Machine Learning - Basic Concepts
- Machine Learning - Python Libraries
- Machine Learning - Applications
- Machine Learning - Life Cycle
- Machine Learning - Required Skills
- Machine Learning - Implementation
- Machine Learning - Challenges & Common Issues
- Machine Learning - Limitations
- Machine Learning - Reallife Examples
- Machine Learning - Data Structure
- Machine Learning - Mathematics
- Machine Learning - Artificial Intelligence
- Machine Learning - Neural Networks
- Machine Learning - Deep Learning
- Machine Learning - Getting Datasets
- Machine Learning - Categorical Data
- Machine Learning - Data Loading
- Machine Learning - Data Understanding
- Machine Learning - Data Preparation
- Machine Learning - Models
- Machine Learning - Supervised
- Machine Learning - Unsupervised
- Machine Learning - Semi-supervised
- Machine Learning - Reinforcement
- Machine Learning - Supervised vs. Unsupervised
- Machine Learning Data Visualization
- Machine Learning - Data Visualization
- Machine Learning - Histograms
- Machine Learning - Density Plots
- Machine Learning - Box and Whisker Plots
- Machine Learning - Correlation Matrix Plots
- Machine Learning - Scatter Matrix Plots
- Statistics for Machine Learning
- Machine Learning - Statistics
- Machine Learning - Mean, Median, Mode
- Machine Learning - Standard Deviation
- Machine Learning - Percentiles
- Machine Learning - Data Distribution
- Machine Learning - Skewness and Kurtosis
- Machine Learning - Bias and Variance
- Machine Learning - Hypothesis
- Regression Analysis In ML
- Machine Learning - Regression Analysis
- Machine Learning - Linear Regression
- Machine Learning - Simple Linear Regression
- Machine Learning - Multiple Linear Regression
- Machine Learning - Polynomial Regression
- Classification Algorithms In ML
- Machine Learning - Classification Algorithms
- Machine Learning - Logistic Regression
- Machine Learning - K-Nearest Neighbors (KNN)
- Machine Learning - Naïve Bayes Algorithm
- Machine Learning - Decision Tree Algorithm
- Machine Learning - Support Vector Machine
- Machine Learning - Random Forest
- Machine Learning - Confusion Matrix
- Machine Learning - Stochastic Gradient Descent
- Clustering Algorithms In ML
- Machine Learning - Clustering Algorithms
- Machine Learning - Centroid-Based Clustering
- Machine Learning - K-Means Clustering
- Machine Learning - K-Medoids Clustering
- Machine Learning - Mean-Shift Clustering
- Machine Learning - Hierarchical Clustering
- Machine Learning - Density-Based Clustering
- Machine Learning - DBSCAN Clustering
- Machine Learning - OPTICS Clustering
- Machine Learning - HDBSCAN Clustering
- Machine Learning - BIRCH Clustering
- Machine Learning - Affinity Propagation
- Machine Learning - Distribution-Based Clustering
- Machine Learning - Agglomerative Clustering
- Dimensionality Reduction In ML
- Machine Learning - Dimensionality Reduction
- Machine Learning - Feature Selection
- Machine Learning - Feature Extraction
- Machine Learning - Backward Elimination
- Machine Learning - Forward Feature Construction
- Machine Learning - High Correlation Filter
- Machine Learning - Low Variance Filter
- Machine Learning - Missing Values Ratio
- Machine Learning - Principal Component Analysis
- Machine Learning Miscellaneous
- Machine Learning - Performance Metrics
- Machine Learning - Automatic Workflows
- Machine Learning - Boost Model Performance
- Machine Learning - Gradient Boosting
- Machine Learning - Bootstrap Aggregation (Bagging)
- Machine Learning - Cross Validation
- Machine Learning - AUC-ROC Curve
- Machine Learning - Grid Search
- Machine Learning - Data Scaling
- Machine Learning - Train and Test
- Machine Learning - Association Rules
- Machine Learning - Apriori Algorithm
- Machine Learning - Gaussian Discriminant Analysis
- Machine Learning - Cost Function
- Machine Learning - Bayes Theorem
- Machine Learning - Precision and Recall
- Machine Learning - Adversarial
- Machine Learning - Stacking
- Machine Learning - Epoch
- Machine Learning - Perceptron
- Machine Learning - Regularization
- Machine Learning - Overfitting
- Machine Learning - P-value
- Machine Learning - Entropy
- Machine Learning - MLOps
- Machine Learning - Data Leakage
- Machine Learning - Resources
- Machine Learning - Quick Guide
- Machine Learning - Useful Resources
- Machine Learning - Discussion
Machine Learning - Real-Life Examples
Machine learning has been transforming various industries by automating processes, predicting outcomes, and discovering patterns in large data sets. Here we are providing the top 5 real-life examples of machine learning −
Fraud Detection in Banking and Finance
Machine learning algorithms are widely used in the financial industry to detect fraudulent activities. These algorithms can analyze transaction data and identify patterns that indicate fraud. For example, credit card companies use machine learning to identify transactions that are likely to be fraudulent and notify customers in real-time. Banks also use machine learning to detect money laundering, identify unusual behavior in accounts, and analyze credit risk.
Healthcare Diagnosis and Treatment
Machine learning algorithms can analyze medical data, such as X-rays, MRI scans, and genomic data, to assist with the diagnosis of diseases. These algorithms can also be used to identify the most effective treatment for a patient based on their medical history and genetic makeup. For example, IBM's Watson for Oncology uses machine learning to analyze medical records and recommend personalized cancer treatments.
Natural Language Processing in Virtual Assistants and Chatbots
Natural language processing (NLP) is an area of machine learning that focuses on understanding and generating human language. NLP is used in virtual assistants and chatbots, such as Siri, Alexa, and Google Assistant, to provide personalized and conversational experiences. Machine learning algorithms can analyze language patterns and respond to user queries in a natural and accurate way.
Autonomous Vehicles
Autonomous vehicles use machine learning algorithms to navigate and make decisions on the road. These algorithms can analyze data from sensors and cameras to identify obstacles and make decisions about how to respond. Autonomous vehicles are expected to revolutionize transportation by reducing accidents and increasing efficiency. Companies such as Tesla, Waymo, and Uber are using machine learning to develop self-driving cars.
Personalized Recommendations in e-commerce and Entertainment
E-commerce platforms, such as Amazon and Netflix, use machine learning algorithms to provide personalized recommendations to users based on their browsing and viewing history. These recommendations can improve customer satisfaction and increase sales. Machine learning algorithms can analyze large amounts of data to identify patterns and predict user preferences, enabling e-commerce platforms and entertainment providers to offer a more personalized experience to their users.
In addition to these examples, machine learning is being used in many other applications, such as energy management, social media analysis, and predictive maintenance. Machine learning is a powerful tool that has the potential to revolutionize many industries and improve the lives of people around the world.
To Continue Learning Please Login