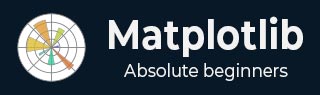
- Matplotlib Basics
- Matplotlib - Home
- Matplotlib - Introduction
- Matplotlib - Vs Seaborn
- Matplotlib - Environment Setup
- Matplotlib - Anaconda distribution
- Matplotlib - Jupyter Notebook
- Matplotlib - Pyplot API
- Matplotlib - Simple Plot
- Matplotlib - Saving Figures
- Matplotlib - Markers
- Matplotlib - Figures
- Matplotlib - Styles
- Matplotlib - Legends
- Matplotlib - Colors
- Matplotlib - Colormaps
- Matplotlib - Colormap Normalization
- Matplotlib - Choosing Colormaps
- Matplotlib - Colorbars
- Matplotlib - Text
- Matplotlib - Text properties
- Matplotlib - Subplot Titles
- Matplotlib - Images
- Matplotlib - Image Masking
- Matplotlib - Annotations
- Matplotlib - Arrows
- Matplotlib - Fonts
- Matplotlib - What are Fonts?
- Setting Font Properties Globally
- Matplotlib - Font Indexing
- Matplotlib - Font Properties
- Matplotlib - Scales
- Matplotlib - Linear and Logarthmic Scales
- Matplotlib - Symmetrical Logarithmic and Logit Scales
- Matplotlib - LaTeX
- Matplotlib - What is LaTeX?
- Matplotlib - LaTeX for Mathematical Expressions
- Matplotlib - LaTeX Text Formatting in Annotations
- Matplotlib - PostScript
- Enabling LaTex Rendering in Annotations
- Matplotlib - Mathematical Expressions
- Matplotlib - Animations
- Matplotlib - Artists
- Matplotlib - Styling with Cycler
- Matplotlib - Paths
- Matplotlib - Path Effects
- Matplotlib - Transforms
- Matplotlib - Ticks and Tick Labels
- Matplotlib - Radian Ticks
- Matplotlib - Dateticks
- Matplotlib - Tick Formatters
- Matplotlib - Tick Locators
- Matplotlib - Axis Ranges
- Matplotlib - Axis Scales
- Matplotlib - Axis Ticks
- Matplotlib - Formatting Axes
- Matplotlib - Axes Class
- Matplotlib - Twin Axes
- Matplotlib - Figure Class
- Matplotlib - Multiplots
- Matplotlib - Grids
- Matplotlib - Object-oriented Interface
- Matplotlib - PyLab module
- Matplotlib - Subplots() Function
- Matplotlib - Subplot2grid() Function
- Matplotlib - Anchored Artists
- Matplotlib - Manual Contour
- Matplotlib - Coords Report
- Matplotlib - AGG filter
- Matplotlib - Ribbon Box
- Matplotlib - Fill Spiral
- Matplotlib - Findobj Demo
- Matplotlib - Hyperlinks
- Matplotlib - Image Thumbnail
- Matplotlib - Plotting with Keywords
- Matplotlib - Create Logo
- Matplotlib - Multipage PDF
- Matplotlib - Multiprocessing
- Matplotlib - Print Stdout
- Matplotlib - Compound Path
- Matplotlib - Sankey Class
- Matplotlib - MRI with EEG
- Matplotlib - Stylesheets
- Matplotlib - Background Colors
- Matplotlib - Basemap
- Matplotlib Event Handling
- Matplotlib - Event Handling
- Matplotlib - Close Event
- Matplotlib - Mouse Move
- Matplotlib - Click Events
- Matplotlib - Scroll Event
- Matplotlib - Keypress Event
- Matplotlib - Pick Event
- Matplotlib - Looking Glass
- Matplotlib - Path Editor
- Matplotlib - Poly Editor
- Matplotlib - Timers
- Matplotlib - Viewlims
- Matplotlib - Zoom Window
- Matplotlib Plotting
- Matplotlib - Bar Graphs
- Matplotlib - Histogram
- Matplotlib - Pie Chart
- Matplotlib - Scatter Plot
- Matplotlib - Box Plot
- Matplotlib - Violin Plot
- Matplotlib - Contour Plot
- Matplotlib - 3D Plotting
- Matplotlib - 3D Contours
- Matplotlib - 3D Wireframe Plot
- Matplotlib - 3D Surface Plot
- Matplotlib - Quiver Plot
- Matplotlib Useful Resources
- Matplotlib - Quick Guide
- Matplotlib - Useful Resources
- Matplotlib - Discussion
Matplotlib - Contour Plots
A contour plot, also known as a contour map or a level plot, is a graphical representation of a three-dimensional surface on a two-dimensional plane.
In a contour plot, the surface is represented by a series of contour lines. Each contour line connects points of equal value on the surface, showing regions where the function has the same value. These contour lines are drawn at constant intervals or "levels", hence the name "level plot".
Imagine you have a contour plot of temperature across a map. Each contour line represents areas with the same temperature, like 50°F, 60°F, and so on. By looking at the plot, you can easily see where it is hotter or cooler across the map −

Contour Plot in Matplotlib
You can create contour plots in Matplotlib using the contour() function in the "matplotlib.pyplot" module. This function accepts X and Y coordinates as either 1D or 2D arrays, representing the grid on which the function "Z" is evaluated. "Z" is a 2D array containing the function values corresponding to the grid points defined by X and Y.
Let's start by drawing a basic contour plot.
Basic Contour Plot
A basic 3D contour in Matplotlib shows contour lines that connect points of equal value, representing the levels or "heights" of the data. Each contour line corresponds to a specific value, forming a map-like representation of the dataset.
Example
In the following example, we are create a basic contour plot. We define the x and y coordinates for the grid, and then use a mathematical function to generate the z values. With these x, y, and z values, we create a contour plot using the contour() function −
import matplotlib.pyplot as plt import numpy as np # Generating data x = np.linspace(-2, 2, 100) y = np.linspace(-2, 2, 100) X, Y = np.meshgrid(x, y) Z = np.sin(X) * np.cos(Y) # Creating contour plot plt.contour(X, Y, Z) # Adding labels and title plt.xlabel('X-axis') plt.ylabel('Y-axis') plt.title('Basic Contour Plot') # Displaying the plot plt.show()
Output
Following is the output of the above code −

Filled Contour Plot
In a filled contour plot in Matplotlib, instead of just showing contour lines, it fills in the areas between the lines with colors, creating a shaded representation of the data surface. Each color represents a different level or "height" of the data, allowing you to easily see the distribution within the dataset.
Example
In here, we create a filled contour plot using the contourf() function, which colors the regions between contour lines −
import matplotlib.pyplot as plt import numpy as np # Generating data x = np.linspace(-2, 2, 100) y = np.linspace(-2, 2, 100) X, Y = np.meshgrid(x, y) Z = np.sin(X) * np.cos(Y) # Creating a filled contour plot plt.contourf(X, Y, Z) # Adding labels and title plt.xlabel('X-axis') plt.ylabel('Y-axis') plt.title('Filled Contour Plot') # Displaying the plot plt.show()
Output
On executing the above code we will get the following output −

Contour Plot with Specific Levels
In a contour plot with specific levels, you specify the levels at which you want the contours to be drawn. Each contour line connects points of equal value, representing different levels or "heights" of the data. This allows you to customize the visualization to highlight specific features or intervals within the dataset.
Example
In this example, we customize the contour plot by specifying specific contour levels using Matplotlib. After generating the data and creating the contour plot, we use the levels parameter in the contour() function to define the contour levels −
import matplotlib.pyplot as plt import numpy as np # Generating data x = np.linspace(-2, 2, 100) y = np.linspace(-2, 2, 100) X, Y = np.meshgrid(x, y) Z = np.sin(X) * np.cos(Y) # Defining contour levels levels = np.linspace(-1, 1, 20) # Creating contour plot with specific levels plt.contour(X, Y, Z, levels=levels) # Adding labels and title plt.xlabel('X-axis') plt.ylabel('Y-axis') plt.title('Contour Plot with Specific Levels') # Displaying the plot plt.show()
Output
After executing the above code, we get the following output −

Contour Plot with Colorbar
In Matplotlib, a contour plot with a colorbar displays contour lines to show points of equal value in the dataset, and a colorbar alongside the plot to indicate the correspondence between colors and data values. The colorbar acts as a visual guide, helping you to understand the range and distribution of data values represented by different colors in the plot.
Example
Here, we create a contour plot with a colorbar using Matplotlib. After generating the data and creating the contour plot, we add a colorbar to the plot using the colorbar() function. This colorbar provides a visual representation of the z values corresponding to the contour plot −
import matplotlib.pyplot as plt import numpy as np # Generating data x = np.linspace(-2, 2, 100) y = np.linspace(-2, 2, 100) X, Y = np.meshgrid(x, y) Z = np.sin(X) * np.cos(Y) # Creating a contour plot contour = plt.contour(X, Y, Z) # Adding colorbar plt.colorbar(contour, label='Z-values') # Adding labels and title plt.xlabel('X-axis') plt.ylabel('Y-axis') plt.title('Contour Plot with Colorbar') # Displaying the plot plt.show()
Output
On executing the above code we will get the following output −

To Continue Learning Please Login