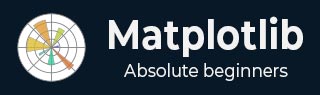
- Matplotlib Basics
- Matplotlib - Home
- Matplotlib - Introduction
- Matplotlib - Vs Seaborn
- Matplotlib - Environment Setup
- Matplotlib - Anaconda distribution
- Matplotlib - Jupyter Notebook
- Matplotlib - Pyplot API
- Matplotlib - Simple Plot
- Matplotlib - Saving Figures
- Matplotlib - Markers
- Matplotlib - Figures
- Matplotlib - Styles
- Matplotlib - Legends
- Matplotlib - Colors
- Matplotlib - Colormaps
- Matplotlib - Colormap Normalization
- Matplotlib - Choosing Colormaps
- Matplotlib - Colorbars
- Matplotlib - Text
- Matplotlib - Text properties
- Matplotlib - Subplot Titles
- Matplotlib - Images
- Matplotlib - Image Masking
- Matplotlib - Annotations
- Matplotlib - Arrows
- Matplotlib - Fonts
- Matplotlib - What are Fonts?
- Setting Font Properties Globally
- Matplotlib - Font Indexing
- Matplotlib - Font Properties
- Matplotlib - Scales
- Matplotlib - Linear and Logarthmic Scales
- Matplotlib - Symmetrical Logarithmic and Logit Scales
- Matplotlib - LaTeX
- Matplotlib - What is LaTeX?
- Matplotlib - LaTeX for Mathematical Expressions
- Matplotlib - LaTeX Text Formatting in Annotations
- Matplotlib - PostScript
- Enabling LaTex Rendering in Annotations
- Matplotlib - Mathematical Expressions
- Matplotlib - Animations
- Matplotlib - Artists
- Matplotlib - Styling with Cycler
- Matplotlib - Paths
- Matplotlib - Path Effects
- Matplotlib - Transforms
- Matplotlib - Ticks and Tick Labels
- Matplotlib - Radian Ticks
- Matplotlib - Dateticks
- Matplotlib - Tick Formatters
- Matplotlib - Tick Locators
- Matplotlib - Axis Ranges
- Matplotlib - Axis Scales
- Matplotlib - Axis Ticks
- Matplotlib - Formatting Axes
- Matplotlib - Axes Class
- Matplotlib - Twin Axes
- Matplotlib - Figure Class
- Matplotlib - Multiplots
- Matplotlib - Grids
- Matplotlib - Object-oriented Interface
- Matplotlib - PyLab module
- Matplotlib - Subplots() Function
- Matplotlib - Subplot2grid() Function
- Matplotlib - Anchored Artists
- Matplotlib - Manual Contour
- Matplotlib - Coords Report
- Matplotlib - AGG filter
- Matplotlib - Ribbon Box
- Matplotlib - Fill Spiral
- Matplotlib - Findobj Demo
- Matplotlib - Hyperlinks
- Matplotlib - Image Thumbnail
- Matplotlib - Plotting with Keywords
- Matplotlib - Create Logo
- Matplotlib - Multipage PDF
- Matplotlib - Multiprocessing
- Matplotlib - Print Stdout
- Matplotlib - Compound Path
- Matplotlib - Sankey Class
- Matplotlib - MRI with EEG
- Matplotlib - Stylesheets
- Matplotlib - Background Colors
- Matplotlib - Basemap
- Matplotlib Event Handling
- Matplotlib - Event Handling
- Matplotlib - Close Event
- Matplotlib - Mouse Move
- Matplotlib - Click Events
- Matplotlib - Scroll Event
- Matplotlib - Keypress Event
- Matplotlib - Pick Event
- Matplotlib - Looking Glass
- Matplotlib - Path Editor
- Matplotlib - Poly Editor
- Matplotlib - Timers
- Matplotlib - Viewlims
- Matplotlib - Zoom Window
- Matplotlib Plotting
- Matplotlib - Bar Graphs
- Matplotlib - Histogram
- Matplotlib - Pie Chart
- Matplotlib - Scatter Plot
- Matplotlib - Box Plot
- Matplotlib - Violin Plot
- Matplotlib - Contour Plot
- Matplotlib - 3D Plotting
- Matplotlib - 3D Contours
- Matplotlib - 3D Wireframe Plot
- Matplotlib - 3D Surface Plot
- Matplotlib - Quiver Plot
- Matplotlib Useful Resources
- Matplotlib - Quick Guide
- Matplotlib - Useful Resources
- Matplotlib - Discussion
Matplotlib - Dateticks
In general plotting, dateticks refer to the labels of the tick lines or markers on the axes of a plot with dates or times, replacing the default numeric values. This feature is especially useful when dealing with time series data.
The image below illustrates Dateticks on a plot −

Dateticks in Matplotlib
Matplotlib provides powerful tools for plotting time-series data, allowing users to represent dates or times instead of the default numeric values on the tick lines or markers (dateticks). The library simplifies the process of working with dates by converting date instances into days since the default epoch (1970-01-01T00:00:00). This conversion, along with tick locating and formatting, occurs in the background, making it transparent to the user.
The matplotlib.dates module plays a key role in handling various date-related functionalities. This includes converting data to datetime objects, formatting dateticks labels, and setting the frequency of ticks.
Basic Dateticks with DefaultFormatter
Matplotlib sets the default tick locator and formatter for the axis, using the AutoDateLocator and AutoDateFormatter classes respectively.
Example
This example demonstrates the plotting of time-series data, here Matplotlib handles the date formatting automatically.
import numpy as np import matplotlib.pylab as plt # Generate an array of dates times = np.arange(np.datetime64('2023-01-02'), np.datetime64('2024-02-03'), np.timedelta64(75, 'm')) # Generate random data for y axis y = np.random.randn(len(times)) # Create subplots fig, ax = plt.subplots(figsize=(7,4), facecolor='.9') ax.plot(times, y) ax.set_xlabel('Dataticks',color='xkcd:crimson') ax.set_ylabel('Random data',color='xkcd:black') plt.show()
Output
On executing the above code we will get the following output −

Customizing Dateticks with DateFormatter
For manual customization of date formats, Matplotlib provides the DateFormatter module, which allows users to change the format of dateticks.
Example
This example demonstrates how to manually customize the format of Dateticks.
import numpy as np import matplotlib.pylab as plt import matplotlib.dates as mdates # Generate an array of dates times = np.arange(np.datetime64('2023-01-02'), np.datetime64('2024-02-03'), np.timedelta64(75, 'm')) # Generate random data for y axis y = np.random.randn(len(times)) # Create subplots fig, ax = plt.subplots(figsize=(7,5)) ax.plot(times, y) ax.set_title('Customizing Dateticks using DateFormatter') ax.xaxis.set_major_formatter(mdates.DateFormatter('%Y-%b')) # Rotates and right-aligns the x labels so they don't overlap each other. for label in ax.get_xticklabels(which='major'): label.set(rotation=30, horizontalalignment='right') plt.show()
Output
On executing the above code we will get the following output −

Advanced Formatting with ConciseDateFormatter
The ConciseDateFormatter class in Matplotlib simplifies and enhances the appearance of dateticks. This formatter is designed to optimize the choice of strings for tick labels and minimizes their length, which often removes the need to rotate the labels.
Example
This example uses the ConciseDateFormatter and AutoDateLocator classes to set the best tick limits and date formats for the plotting.
import datetime import matplotlib.pyplot as plt import matplotlib.dates as mdates import numpy as np # Define a starting date base_date = datetime.datetime(2023, 12, 31) # Generate an array of dates with a time delta of 2 hours num_hours = 732 dates = np.array([base_date + datetime.timedelta(hours=(2 * i)) for i in range(num_hours)]) date_length = len(dates) # Generate random data for the y-axis np.random.seed(1967801) y_axis = np.cumsum(np.random.randn(date_length)) # Define different date ranges date_ranges = [ (np.datetime64('2024-01'), np.datetime64('2024-03')), (np.datetime64('2023-12-31'), np.datetime64('2024-01-31')), (np.datetime64('2023-12-31 23:59'), np.datetime64('2024-01-01 13:20')) ] # Create subplots for each date range figure, axes = plt.subplots(3, 1, constrained_layout=True, figsize=(6, 6)) for nn, ax in enumerate(axes): # AutoDateLocator and ConciseDateFormatter for date formatting locator = mdates.AutoDateLocator(minticks=3, maxticks=7) formatter = mdates.ConciseDateFormatter(locator) ax.xaxis.set_major_locator(locator) ax.xaxis.set_major_formatter(formatter) # Plot the random data within the specified date range ax.plot(dates, y_axis) ax.set_xlim(date_ranges[nn]) axes[0].set_title('Concise Date Formatter') # Show the plot plt.show()
Output
On executing the above code we will get the following output −

To Continue Learning Please Login